Developing Healthcare in Resource-Poor Countries through AI
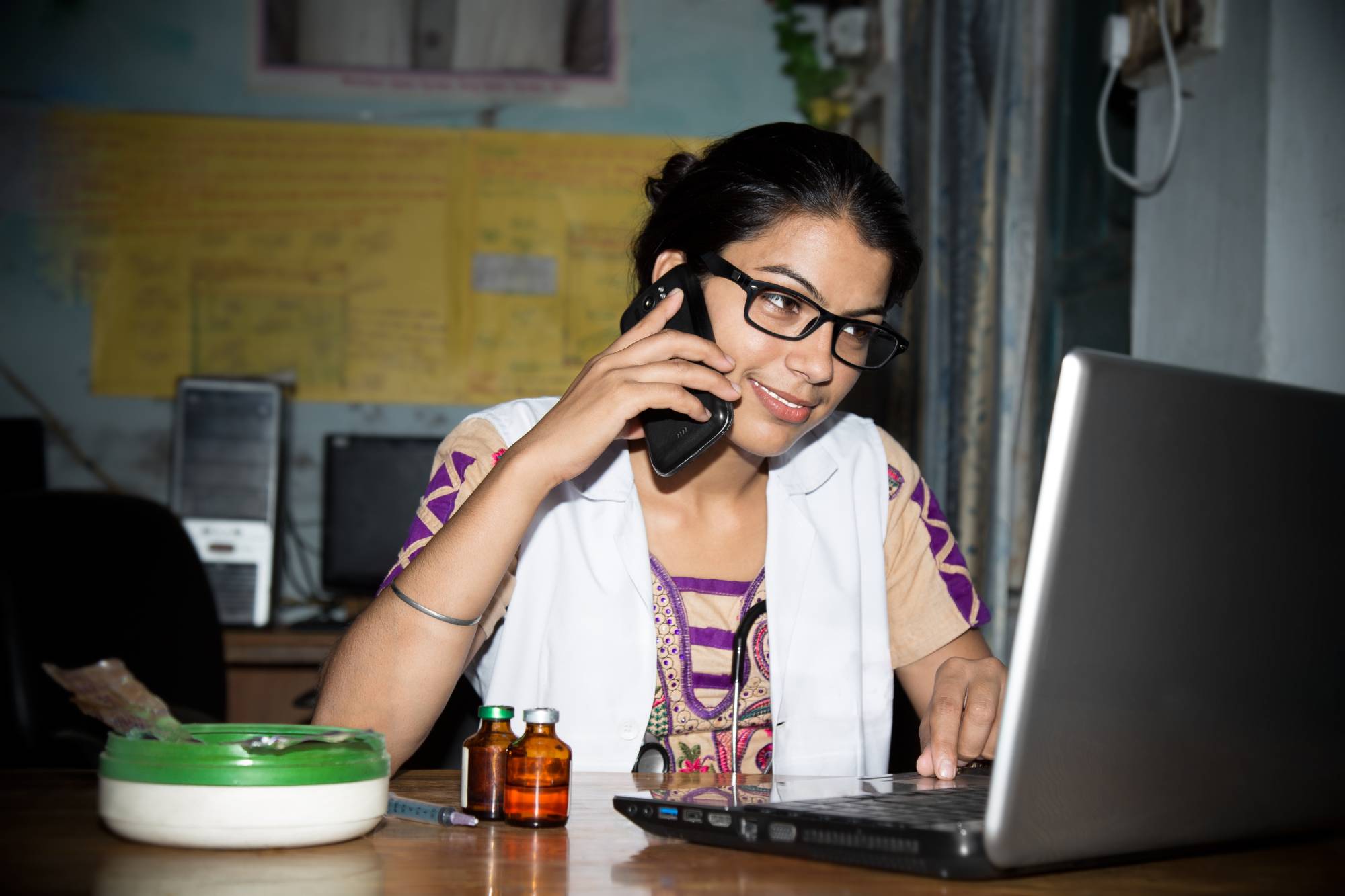
As we observe artificial intelligence (AI) extending its reach into many interdisciplinary fields (well beyond that of just the technology industry), one area that shows great promise is AI’s application to healthcare, focused specifically on resource-poor countries. When exploring AI’s capacity to improve patient care within these countries, it is crucial to recognize that there are multiple kinds of AI with various uses. Utilizing these various AI tools and strategies in a growing number of resource-poor countries, ventures such as the global health initiatives (GHI) promote systems of self-sustainable healthcare. However, it is critical to stress test security barriers for patient privacy, as well as consider ethical implications for patient well-being.
The two main types of AI are artificial general intelligence, which mimics the human brain’s ability to perform and adapt in significantly different scenarios, and artificial narrow intelligence, the ability of a machine to do one task extremely well [1]. In addition, there are many subfields that can be blended in a single application, which include expert systems, machine learning, natural language processing (NLP), automated planning and scheduling, and image and signal processing. These functions have been incorporated into numerous proposed solutions addressing questions from improving medical testing capability and capacity, to creating readily available mobile applications, all aimed towards enabling resource-poor settings to achieve “an initial diagnosis at a remarkably low cost,” [2]. With the growth of such research, AI will likely become even more valuable. Further, AI will only improve as GHIs focus on promoting a model of self-sustainability. While it is understood that the introduction of AI will likely be provided through international support based outside of these developing countries, there is emphasis on the idea that there should be a transition of the assets directly in the region of interest. This will include the “training of local healthcare providers”, acknowledging infrastructure restraints, and adapting to new technological model features [3].
The role of data and its limitations is another decisive factor when developing and using such technology. In supervised machine learning, for instance, these kinds of “applications require high-quality datasets that can be used to train machine learning algorithms to identify risk factors or make disease diagnoses” [1]. However, considering that the goal is to implement AI in developing countries, it is difficult and time-consuming to acquire the necessary data to construct an accurate model. In addition, “probing models that can decrypt the data and pick features that can lead to disease prediction will play a pivotal role in inching closer to personalized medicine” [4]. Data is one of the main reasons that AI can be highly effective, but data also becomes one of the biggest challenges to AI applications in resource-poor settings.
This field of technology, so heavily reliant on information, cannot be discussed without properly addressing its ethical and security implications. While many research teams striving for transparency have already proposed they release all models used to create their algorithms, it is imperative that they address any biases that may come up as a result of the data. For instance, when AI was used to calculate the risk of a criminal reoffending, there was a reported bias against black citizens [1]. Recognizing these kinds of unintended prejudices is significant, as doing so will prevent AI from harming already vulnerable populations. Sharing machine learning models is also very important so that other researchers can access them to compare their own findings and further improve health outcomes [5]. The act of making the models public not only benefits researchers, but also policymakers as they are able to examine them and make sure the practices remain within guidelines concerning consent, privacy, and data ownership [5].
References
[1] Wahl, Brian et al. “Artificial intelligence (AI) and global health: how can AI contribute to health in resource-poor settings?” BMJ Global Health vol. 3,4 e000798. 29 Aug. 2018, ;doi:10.1136/bmjgh-2018-000798
[2] Melander, David et al. “Diagnostics as the Key to Advances in Global Health: Proposed Methods for Making Reliable Diagnostics Widely Available.” Journal of Medical Devices vol.14,1 (2020): 014702. doi:10.1115/1.4046046. https://www.ncbi.nlm.nih.gov/pmc/articles/PMC7164509/
[3] Hadley, Trevor D et al. “Artificial Intelligence in Global Health - A Framework and Strategy for Adoption and Sustainability.” International Journal of Maternal Child Health and AIDS vol. 9,1 (2020): 121-127. doi:10.21106/ijma.296
[4] Thomford, Nicholas E et al. “Implementing Artificial Intelligence and Digital Health in Resource-Limited Settings? Top 10 Lessons We Learned in Congenital Heart Defects and Cardiology.” OMICS: A Journal of Integrative Biology vol. 24,5 (2020): 264-277. doi:10.1089/omi.2019.0142
[5] Carrillo-Larco, Rodrigo M et al. “Machine learning health-related applications in low-income and middle-income countries: a scoping review protocol.” BMJ Open vol. 10,5 e035983. 10 May 2020, doi:10.1136/bmjopen-2019-035983