AI and the Future of Electronic Health Records
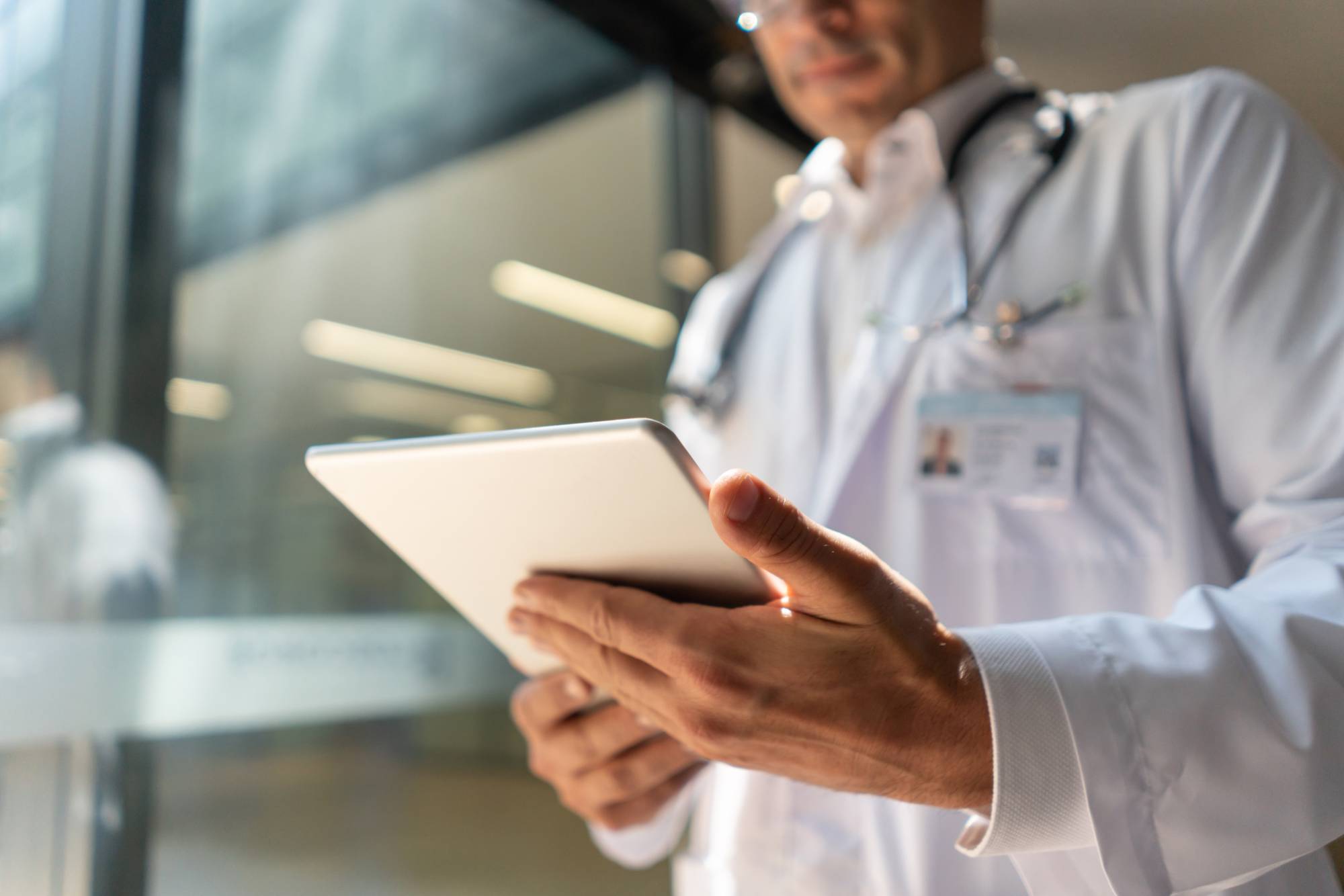
Artificial intelligence (AI) is human-level intelligence displayed by machines (e.g. computers). One subset of AI is machine learning, the study of algorithms that improve from experience [1]. These algorithms must be “trained” to recognize certain patterns, outcomes, or features within a dataset. Another subset of AI is natural language processing, the study of algorithms that can interpret human-generated language data [1]. There is some overlap between machine learning and natural language processing, along with other subsets of AI (e.g. deep learning).
In the healthcare setting, data comes in many forms, like medical imaging and patient records. To use AI, one needs digitized data, which presents an issue particularly with paper records. Electronic medical records (EMRs) digitize the paper charts often used in hospitals, clinics, and other settings [2]. More broadly, electronic health records (EHRs) consolidate data from multiple clinicians and other sources; an EHR may contain multiple EMRs [2].
Once a patient’s EHR is developed or curated (either by manual data entry or automated scanning methods), AI can help analyze the data within. For instance, an NLP-based recommendation system can analyze the written notes of clinicians, sifting through words, phrases, sentences, and other language “tokens” to identify whether the patient is at risk of disease. If the system recommends the patient for follow-up care or specialized screening, its analysis amounts to an early diagnosis, which may be lifesaving [3]. Such methods already exist for assessing the likelihood of heart failure [4] and breast cancer recurrence [5], and future research will address more conditions.
Some patients are asymptomatic, meaning that data from a clinician’s notes would not necessarily suggest disease. To benefit patient outcomes in this scenario, the EHR of the future must include cutting-edge data sources. For instance, an AI algorithm could analyze data from a whole genome sequence and evaluate a patient’s current health or future health prospects [6]. The cost of obtaining a person’s whole genome sequence has fallen to roughly $1,000 as of 2019, making it more accessible for EHRs [7]. With additional data on the human proteome, microbiome, and other sources, EHRs also open the door to “multi-omics” data analysis [6]. Applications include personalized medicine, a branch of healthcare where genomic and other data are essential for success [6].
In addition to benefiting individual patients, EHRs can also assist public health and research across populations. An example of a public health dataset is the Big Cities Health Inventory, which contains “over 18,000 datapoints” from “30 of the largest, most urban cities in the United States” [8]. Although 18,000 datapoints sounds large, it is a small fraction of the millions of people living in these 30 cities. Using over 270,000 EMRs, a study used machine learning algorithms to build a “knowledge graph,” connecting diseases to symptoms in a large graph structure [9]. These tools can be used to monitor entire cities, tracking public health in near-real time. Combined with AI recommendation systems and predictive algorithms, public health practitioners could build and execute a data-driven plan of action even during the largest crises.
In summary, the future of EHRs is one of big data at the patient and population level, with access to cutting-edge data sources and data analysis tools. However, the promises of patient outcomes and public health come with privacy and security concerns. Patient consent for enrollment, patient control over their EHR’s contents, and special protections for “sensitive information” like mental health history and substance abuse, are just three of many considerations [10]. Technical methods like decentralized machine learning and other tools should be combined with sound healthcare policy in order to facilitate the safe and rapid uptake of EHRs.
References
[1] Jiang F, Jiang Y, Zhi H, et al. Artificial Intelligence in Healthcare: Past, Present and Future. Stroke and Vascular Neurology 2017; 2: e000101. DOI:10.1136/svn-2017-000101.
[2] What are the Differences between Electronic Medical Records, Electronic Health Records, and Personal Health Records? (n.d.). Retrieved July 14, 2020, from https://www.healthit.gov/faq/what-are-differences-between-electronic-medical-records-electronic-health-records-and-personal.
[3] Névéol A, and Zweigenbaum P. Clinical Natural Language Processing in 2014: Foundational Methods Supporting Efficient Healthcare. Yearbook of Medical Informatics 2015; 10: 194-8. DOI:10.15265/IY-2015-035.
[4] Vijayakrishnan R, Steinhubl S, Ng K, et al. Prevalence of Heart Failure Signs and Symptoms in a Large Primary Care Population Identified Through the Use of Text and Data Mining of the Electronic Health Record. Journal of Cardiac Failure 2014; 20-7. DOI:10.1016/j.cardfail.2014.03.008.
[5] Carrell D, Halgrim S, Tran D et al. Using Natural Language Processing to Improve Efficiency of Manual Chart Abstraction in Research: The Case of Breast Cancer Recurrence. American Journal of Epidemiology 2014; 179-6. DOI:10.1093/aje/kwt441.
[6] Abul-Husn N, and Kenny E. Personalized Medicine and the Power of Electronic Health Records. Cell 2019; 177. DOI:10.1016/j.cell.2019.02.039.
[7] DNA Sequencing Costs: Data. October 2019. Retrieved July 14, 2020, from https://www.genome.gov/about-genomics/fact-sheets/DNA-Sequencing-Costs-Data.
[8] About Big Cities Health. (n.d.). Retrieved July 14, 2020, from https://www.bigcitieshealth.org/bchi-about.
[9] Rotmensch M, Halpern Y, Tlimat A, et al. Learning a Health Knowledge Graph from Electronic Medical Records. Scientific Reports 2017; 7: 5994.
[10] Rothstein, M. Health Privacy in the Electronic Age. Journal of Legal Medicine 2007; 28: 4. DOI:10.1080/01947640701732148.