The Role of AI in Precision Medicine
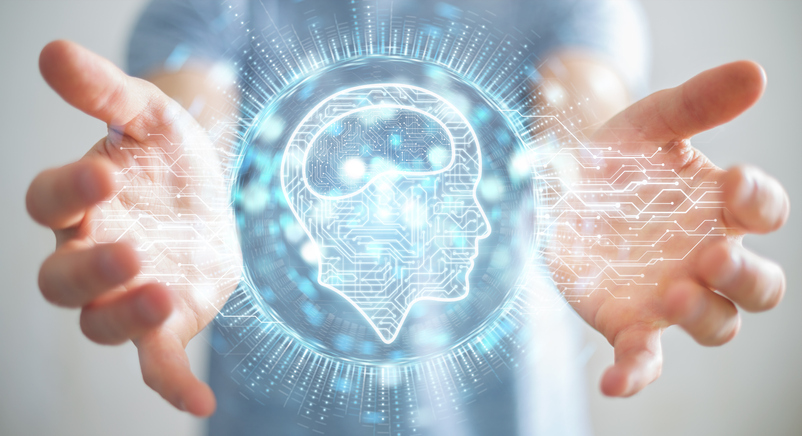
Disruptive technology currently gathers significant influence throughout the spectrum of medicine from biomedical research to clinical practices. Advancements in technology have resulted in greater data capabilities, including data extraction, storage, and analysis, which have in turn led the shift from algorithm-centric to data-centric insights within precision medicine [1]. Furthermore, the field of medicine now undergoes a pivotal moment, as it shifts from empirical medicine in which “one drug/treatment fits all” based on “signs and symptoms” to precision medicine in which patient stratification enables better prevention, early detection, and targeted drugs and treatment for a more homogenous patient population [2].
Artificial intelligence (AI) serves a vital role during this transition as it not only assists computational biology with data mining of multi-omics datasets and medical images, but also provides the field of bioinformatics machine learning (ML) tools, specifically deep learning (DL) tools which “can encode and learn from heterogeneous and complex data, in both supervised and unsupervised settings” [1, 3]. Unsupervised learning tends to be used first for clustering, dimensionality reduction, and feature extraction through popular algorithms, such as k-nearest neighbors (k-nn), in order to identify any key genomic/molecular variables or relationships. Supervised learning, on the other hand, utilizes classification or regression algorithms and compares its predictions with actual lab or clinical data to improve its model [4]. As of 2019, “the most successful application of DL in biomedical research…has been in supervised learning,” indicating that researchers and medical staff must carefully document lab and patient data in order to avoid biases, and empower patients as their informed participation and privacy protection are crucial for success [2, 3].
One key way researchers seek to grow precision medicine through AI includes the development of biomarkers (BMs), which “have been used to improve patient’s stratification and/or develop targeted therapies facilitating the decision-making process throughout the new drug development process” [2]. Using omics datasets like The Cancer Genome Atlas (TCGA) along with popular DL algorithms including convolutional neural network (CNN), deep feedforward neural network (DFF), and autoencoders (AEs), researchers have been able to successfully identify interactive genes, classify different cancer types through methylation patterns, classify cancer types based on somatic mutations, etc.–all of which can serve as potential BMs [3].
New AI-driven BMs yet not only open the gates for new drug discovery, but also empower the approach of drug repositioning (i.e. drug repurposing), resulting in significant time cost reductions. ML approaches to drug repositioning include four main steps: compound identification (finds candidate drug for alternative molecular targets), compound acquisition (receive license of candidate drug), development (can begin in preclinical (Phase 1) or Phase 2 drug research), and FDA post-market safety monitoring [4]. Additionally, ML approaches can be split into three main categories, network-based that look at interconnections of interest between drugs and diseases, text-mining that utilize published literature, and semantic-based that use prior biomedical knowledge for inspiring the direction of future drug development [4].
Another area researchers and medical staff utilize AI to advance precision medicine is within medical imaging and digital pathology. Already, there are DL image recognition applications that can accurately classify skin lesions, leading to future developments in mobile applications for early detection of skin cancer [3]. Although there were initial setbacks in processing 3-dimensional images and addressing the issue of standardizing data coming from different clinical practices that use different devices and experimental procedures, recent studies have also been able to use DL approaches to work with MRI images, CT scans, ultrasounds, and X-rays [3]. Such collaboration resulted in significant time cost reduction for applications such as tumor segmentation, identification of vessel regions, bone age assessment, etc. [3].
References
[1] Filipp, Fabian V. “Opportunities for Artificial Intelligence in Advancing Precision Medicine.” Current genetic medicine reports vol. 7,4 (2019): 208-213. doi:10.1007/s40142-019-00177-4
[2] Seyhan, Attila A, and Claudio Carini. “Are innovation and new technologies in precision medicine paving a new era in patients centric care?.” Journal of translational medicine vol. 17,1 114. 5 Apr. 2019, doi:10.1186/s12967-019-1864-9
[3] Martorell-Marugán J, Tabik S, Benhammou Y, et al. Deep Learning in Omics Data Analysis and Precision Medicine. In: Husi H, editor. Computational Biology [Internet]. Brisbane (AU): Codon Publications; 2019 Nov 21. Chapter 3. Available from: https://www.ncbi.nlm.nih.gov/books/NBK550335/ doi: 10.15586/computationalbiology.2019.ch3
[4] Maria Koromina, Maria-Theodora Pandi, and George P. Patrinos.OMICS: A Journal of Integrative Biology.Nov 2019.539-548.http://doi.org/10.1089/omi.2019.0151