The Role of Machine Learning in Diagnosing Alzheimer’s Disease
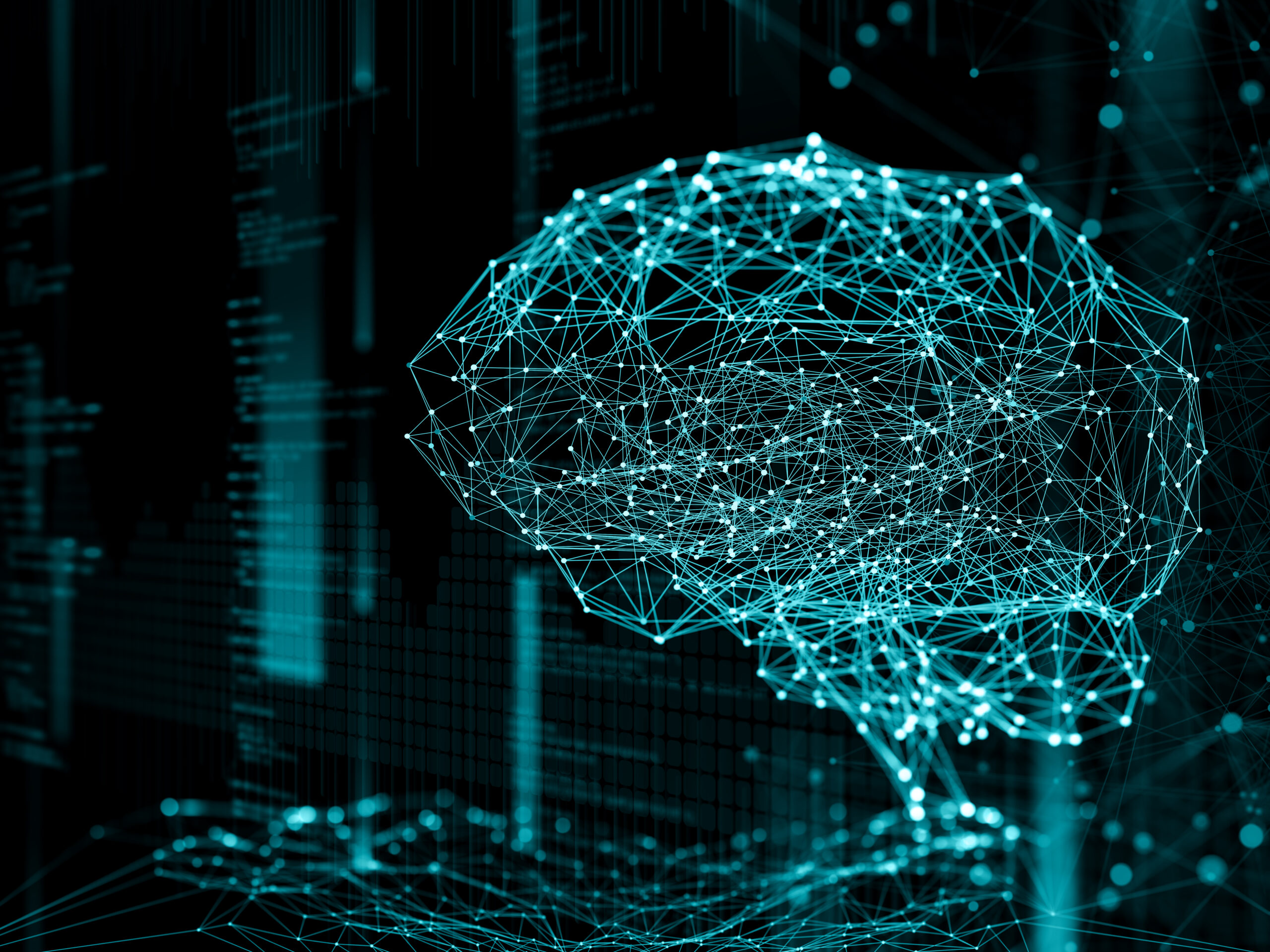
Alzheimer's disease (AD) affects over 35 million people worldwide, and as the population ages, that number is projected to increase over the next decade (Ammar & Ayed, 2018). While there are two FDA-approved drugs that can reduce the effects of the disease, millions of AD patients still suffer from cognitive decline with no treatment options that actually slow the disease's progress from mild cognitive impairment to eventual dementia. It's difficult to even give accurate prognoses to Alzheimer's patients until serious symptoms have appeared. Genetic testing can reveal which individuals are at risk for AD, but some of those individuals may never develop symptoms at all ("Alzheimer Disease," 2018).
Being able to diagnose AD earlier would significantly improve the standard of care for the disease (Sevigny et al., 2016). Furthermore, an earlier diagnosis and prognosis for AD would give patients an earlier start in taking steps to manage the impact of the disease. Elsevier's Clinical Key notes that someone suffering from AD could benefit from more time to emotionally process his situation, build routines, and arrange social circles such that AD would be minimally harmful to the patient and those around (2018). However, prognoses must be based on deeply complex combinations of patient data – many of which are "noise," or irrelevant information that can be challenging to differentiate from more salient factors – and are very difficult for human doctors to provide. For machine learning algorithms (MLAs), however, the problem of accounting for many factors in order to make early diagnoses and prognoses seems far more manageable. MLAs may provide medical professionals with useful analyses based on complex sets of data that account for multiple gene markers, type and history of work, family history, cognitive and neuropsychological task performance, and brain imaging.
MLAs are capable of working with vast sets of data and detecting patterns therein. The power of MLAs can be combined with computational methods of "feature selection" to ensure that MLAs focus only on the most relevant information, and ignore the noise. Researchers at the Monterrey Institute of Technology and Higher Education tested various combinations of feature selection methods and MLA structures on genetic data from the Alzheimer's Disease Neuroimaging Initiative in an effort to benchmark the performance of MLAs in predicting the behavior of Alzheimer's cases. Their findings indicate that machine learning models can diagnose AD with a Receiver Operating Characteristic Area Under the Curve (ROC AUC) of 0.719 (Oriol et al., 2019). ROC AUC provides a useful metric of diagnostic accuracy: an ROC AUC of 0.0 means every diagnosis is incorrect, of 0.5 is equivalent to guessing, and of 1.0 means every diagnosis is correct (Hajian-Tilaki, 2013). The potential effectiveness of machine learning in producing early diagnoses and prognoses of AD is clear. To best leverage that potential, other researchers are investigating which schema yield the most accurate results.
Several gene biomarkers have been tested against each other as potentially helpful sources of MLA training data in a study that found that many markers are not useful, but that myoneurin may be an optimal gene biomarker in MLA-assisted diagnoses moving forward (Ren et al., 2020). Another group of researchers working on genetic data conducted a survey of MLAs' accuracy in predicting the effects of complex interactions between the multiple genes linked to Alzheimer's on the behavior of the disease itself. These interactions, known as epistasis, are better predicted by new software than by previously-designed MLAs. The increasing ability to predict epistasis based on genetic data could be helpful in delivering early diagnoses and prognoses for AD and other complex diseases (Chang et al., 2018). MLAs trained on MRI brain imaging also produced promising results. The most effective models can predict cases with up to 80% accuracy when trained on hippocampal volume data (Uysal & Ozturk, 2019).
A collaborative study between researchers at Catholic University of America and Georgetown University shows that by operating on data sets that combine patients' results on several neuropsychological tests with their performance on a 5.5-minute spatial attention task, a well-designed MLA can predict AD with up to 91.08% predictive accuracy. The study included 28 subjects with AD or cognitive impairment and 50 without. While the MLAs tested were all less effective at correctly recognizing AD patients than correctly recognizing healthy subjects, these results still indicate that training MLAs on task-related data may be effective (Almubark et al., 2019). Analysis of the syntactic, semantic, and pragmatic characteristics of transcriptions of patients' speech could also prove important. Researchers at Sfax University in Tunisia were able to achieve a predictive accuracy of 79% by training MLAs on these analyses (Ammar & Ayed, 2018).
As methods are refined, training machine learning algorithms on data that is relatively cheap to acquire – such as performance in brief tasks and patients' speech – could provide a lower-cost option for generating earlier diagnoses and prognoses for cases of Alzheimer's, which would lead to better quality of life and potential preventative care for future patients.
References
Almubark, I., Chang, L.-C., Nguyen, T., Turner, R. S., & Jiang, X. (2019). Early Detection of Alzheimer’s Disease Using Patient Neuropsychological and Cognitive Data and Machine Learning Techniques. 2019 IEEE International Conference on Big Data (Big Data). doi: 10.1109/bigdata47090.2019.9006583
Alzheimer Disease. (2018, June 14). Retrieved April 15, 2020, from https://www.clinicalkey.com/#!/content/clinical_overview/67-s2.0-b5a1fbe1-e715-49b1-973a-a19b83669f88
Ammar, R. B., & Ayed, Y. B. (2018). Speech Processing for Early Alzheimer Disease Diagnosis: Machine Learning Based Approach. 2018 IEEE/ACS 15th International Conference on Computer Systems and Applications (AICCSA). doi: 10.1109/aiccsa.2018.8612831
Chang, Y.-C., Wu, J.-T., Hong, M.-Y., Tung, Y.-A., Hsieh, P.-H., Yee, S. W., … Chen, C.-Y. (2018). GenEpi: Gene-based Epistasis Discovery Using Machine Learning. doi: 10.1101/421719
Hajian-Tilaki, K. (2013). Receiver Operating Characteristic (ROC) Curve Analysis for Medical Diagnostic Test Evaluation . Caspian Journal of Internal Medicine, 4(2), 627–635. Retrieved from https://www.ncbi.nlm.nih.gov/pmc/articles/PMC3755824/
Mahyoub, M., Randles, M., Baker, T., & Yang, P. (2018). Comparison Analysis of Machine Learning Algorithms to Rank Alzheimer’s Disease Risk Factors by Importance. 2018 11th International Conference on Developments in ESystems Engineering (DeSE). doi: 10.1109/dese.2018.00008
Oriol, J. D. V., Vallejo, E. E., Estrada, K., Peña, J. G. T., & Initiative, T. A. S. D. N. (2019). Benchmarking machine learning models for late-onset alzheimer’s disease prediction from genomic data. BMC Bioinformatics, 20(1). doi: 10.1186/s12859-019-3158-x
Ren, J., Zhang, B., Wei, D., & Zhang, Z. (2020). Identification of Methylated Gene Biomarkers in Patients with Alzheimer’s Disease Based on Machine Learning. BioMed Research International, 2020. doi: 10.1155/2020/8348147
Sevigny, J., Chiao, P., Bussière, T., Weinreb, P. H., Williams, L., Maier, M., … Sandrock, A. (2016). The antibody aducanumab reduces Aβ plaques in Alzheimer’s disease [Abstract]. Nature, 537(7618), 50–56. doi:10.1038/nature19323
Uysal, G., & Ozturk, M. (2019). Using Machine Learning Methods for Detecting Alzheimers Disease through Hippocampal Volume Analysis. 2019 Medical Technologies Congress (TIPTEKNO). doi: 10.1109/tiptekno.2019.8895135
What to know about the stages of Alzheimer's. (2019, April 19). Retrieved April 15, 2020, from https://www.mayoclinic.org/diseases-conditions/alzheimers-disease/in-depth/alzheimers-stages/art-20048448