The Significance of Artificial Intelligence in Drug Delivery Systems
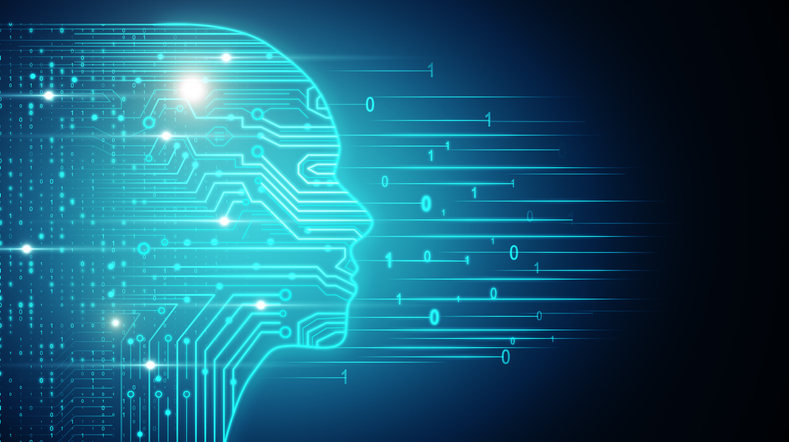
Artificial intelligence (AI), defined by the ability of a machine to simulate human cognitive functions, might be significant within the development of drug delivery systems. Whether by automated workflows/databases through Machine Learning (ML) for rapid analysis or Artificial Neural Networks (ANN), AI can be used in developing predictions and evaluations of drug delivery and can also be directly applied to improving electronic drug delivery systems.
The traditional process of drug delivery systems design is associated with several challenges such as predicting the response to a particular formulation along with the occurrence of unexpected phenomena. Through statistical recognition methods, computational intelligence, biological-based approaches (e.g. neural networks), and probability theories, AI tools could enable the prediction of in vivo response of a given drug.
For example, ML-based data-driven systems could help predict and analyze the trade-off between drug-dosage and effective pathogen killing. Oftentimes, patients begin to take a given drug against a pathogen but feel side effects that deter them from continuing treatment, leading to a potential for a drug resistant strain to emerge. ML systems that are online, unsupervised, and data-driven could ultimately predict the effectiveness of multiple dosing schemes, allowing for reduced experimental costs, and an increased speed of prediction that would better estimate conditions of drug intake. These ML systems could also allow researchers to investigate more effective treatments by evaluating a larger pool of doses than possible with experiments (2).
Aside from ML, ANNs could also be useful to drug delivery systems. ANNs are made up of interconnected processing elements (hardware or algorithms) that process information from an external environment. These interconnected processing elements are created via simulating the network of model neurons that are trained, using past information, to predict the present and future states of a system or to perform highly specific tasks.
ANNs can be directly applied to implantable microchips, for example, which involve electronically-controlled drug release and are promising tools in drug delivery. Within microchips, ANNs could contribute to creating more efficient automatic adjustment of drug concentration as well as controlled timing of drug release. This could be potentially important in chronic diseases which require timely treatment and regular monitoring (3).
The possibilities and applicability of ANNs and ML in drug delivery systems does not come without challenges. High costs of maintenance, repair, frequent upgrading of software, lost code recovery, lack of appropriate response to the altering environment, or ethical concerns, are all potential obstacles. For AI-based models for drug delivery systems, integration of experimental procedures and in silico simulations are also necessary to reduce rates of false negative or positive predictions. Therefore, AI models along with the conventional methods could potentially optimize controlled release drug delivery systems and evaluation of delivery systems.
References:
1. https://www.sciencedirect.com/science/article/abs/pii/S0169409X19300511
2. https://journals.plos.org/plosone/article?id=10.1371/journal.pone.0031724